Completing the Loop - AI for Semiconductor and Systems Design
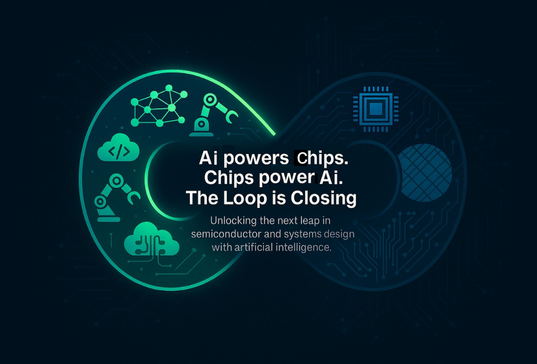
First: Semiconductors for AI
The continued advancement of semiconductor technology is one of the critical drivers behind deployment of large AI models. As models become larger and more intricate, the demand for high-performance hardware grows. Today, innovations in chip architecture including integration of AI-optimized components, lower power consumption, and increased memory bandwidth, are making it possible to run large-scale AI models. This is critical not only for training but also for deploying AI in edge devices, where smaller, faster, and less power-hungry chips can enable AI-driven solutions in areas like autonomous vehicles, healthcare diagnostics, and industrial robotics.
Deployment of AI
As semiconductor technology advances, it directly supports the scalability, flexibility, and accessibility of large AI models across diverse industries. And in turn, the successful deployment of AI in industries like manufacturing, supply chain, and cybersecurity changes the nature of decision-making. Today, AI-driven systems are optimizing production lines, predicting equipment failure through predictive maintenance, and streamlining quality control. In supply chain, AI models are being able to analyse vast amounts of data to forecast demand, optimize inventory, and enhance logistics management, resulting in cost savings and faster delivery times. Similarly, in cybersecurity, AI models are increasingly being deployed to detect and mitigate cyber threats by analyzing patterns and anomalies in real time, enabling quicker responses to emerging security risks.
But we have not come full circle. While advancements in semiconductors have enabled large-scale deployment of AI which has enabled productivity improvements across sectors, the deployment of AI for semiconductor & systems design leaves much to be desired.
The Current Landscape in Semiconductor & Electronics Design
Despite the significant advancements in AI, automation in areas like Integrated Circuit (IC) design and systems design remains limited. So, in essence, the processes that enable AI automation are still largely reliant on manual expertise.
In IC and circuit design, much of the design process involves human intervention to meet specifications, handle trade-offs, and ensure that the design aligns with technology trends. DFM is often a manual, iterative process where engineers consider a myriad of factors, such as material compatibility, component placement, and manufacturability constraints. Similarly, DFMEA which is crucial in identifying potential failure modes and assessing risks in product and process designs, still depends heavily on human-driven expertise. Engineers must spend time evaluating each component and process in the context of its failure consequences.
In summary, the design process is plagued by challenges, including protracted design cycles, limited automation, and a heavy reliance on manual (mostly repetitive) iterations. However, recent breakthroughs in artificial intelligence, particularly advancements in Large Language Models (LLMs) and reinforcement learning have made it possible to change this.
We strongly believe that multiple elements of IC and systems design are perfectly suited for AI automation. We also believe that, unlike in other sectors, this endeavour should be pursed in collaboration and integration with incumbents (traditional EDA players in this case).
Capturing Tribal Knowledge through AI
Take the case of IC design. Any engineer will tell you that it is just as much an art as it is a science, codified not in books but passed down in lessons from the master craftsmen.
“Tribal knowledge” and institutional memory in integrated circuits (IC) and systems design capture years of expertise, lessons learned, and practical insights from engineers who have worked on complex projects. This knowledge, often passed down informally or stored in legacy systems, can be difficult to access and integrate into new projects.
AI can help bridge this gap by leveraging models to mine historical design documents, failure reports, and engineering notes, transforming unstructured data into actionable insights. By analyzing patterns across past projects, AI can identify design trends, best practices, and potential pitfalls, making this tacit knowledge more accessible to newer team members or those working on different parts of the system.
Completing the Loop: AI for Semiconductors
The need for "Completing the Loop" by using AI for semiconductor design, which enables AI deployment, is becoming increasingly critical in the modern technological landscape.
As AI applications continue to advance and proliferate across industries, they place greater demands on the hardware that supports them—particularly semiconductors. And by leveraging AI in semiconductor design, engineers can significantly enhance the efficiency and performance of chips, optimizing them for AI workloads.
A Roadmap for Integrating AI in Semiconductor Design
The design process typically follows four iterative steps: conceive, design & edit, simulate, and review. In this process, the critical phase for engineers is the "conceive" step, where creativity and problem-solving are key to defining the system's architecture and goals. However, the subsequent steps—design & edit, simulate, and review—are ripe for automation with the help of AI to optimize efficiency and reduce human error.
The order of adopting automation should start with "edit," where AI can automatically refine and adjust the design based on specified parameters. Next, the "review" step can be automated to ensure compliance with specifications and standards, followed by AI-driven simulations that test the design under various scenarios. Finally, the most complex phase, "design," can be assisted with AI tools that propose optimized layouts and configurations, but human engineers still retain final oversight.
For instance in case of analog IC design, an AI copilot could optimize transistor sizes in a VCO to hit frequency targets while adjusting for parasitics in the “edit” phase; during “simulate,” it could run Monte Carlo analysis on an LNA to identify gain instability under process variations; and in “review,” it might verify a bandgap reference meets temperature stability specs and DRC rules, flagging mismatch errors—all while supporting on-prem, cloud, or hybrid deployment for flexibility.
AI tools in this space should also be flexible in terms of deployment scenarios including on-prem, cloud, hybrid to enable quick adoption.
Bridging the Engineering Talent Gap and Cutting R&D Costs
The shortage of skilled engineering talent and the growing complexity of integrated circuits (ICs) and systems design have created significant challenges for the semiconductor industry. As the demand for cutting-edge technology skyrockets, the supply of qualified engineers has struggled to keep pace. AI offers a solution by automating routine tasks and optimizing complex design processes, thus reducing the reliance on labour. With AI tools handling mundane tasks, engineers can focus on more strategic aspects of design, effectively overcoming talent shortages and ensuring that development cycles remain efficient and productive.
Additionally, the rising costs of research and development (R&D) are a major concern for companies in the IC and systems design space. Traditional R&D methods often involve high costs associated with extensive prototyping, testing, and iterations, which can delay time-to-market and consume valuable resources. AI technologies can significantly reduce these costs by enabling faster and more accurate simulations, predictive modelling, and design optimizations. They can scale limited expertise by assisting less experienced designers with optimized suggestions and compliance checks, effectively amplifying the capabilities of smaller teams and reducing reliance on scarce design specialists.
Indian AI-EDA Startups: A Unique Competitive Edge
Leveraging India’s vast, cost-efficient talent pool and supportive government initiatives presents a unique opportunity to rapidly innovate and develop AI-driven tools for semiconductor and systems design.
India is home to many skilled semiconductor designers, data scientists, and AI engineers. By tapping into this talent pool, companies can build high-quality, cutting-edge solutions. Additionally, government initiatives such as the Semicon India Programme provide the necessary infrastructure, funding, and policy support to foster innovation in the semiconductor sector.
This combination of technical expertise and government backing can accelerate the development of AI-powered design tools, enabling quicker, more efficient chip design processes that are adaptable to both local needs and the global market's increasing demand for advanced technologies. Finally, India’s role as both a development hub and a growing market can ensure that these AI-driven tools are globally competitive and tailored to the unique requirements of emerging economies.